Empowering GenAI with relevant details about a business to truly enable it to provide value for an organization can be done through supplementing its knowledge. A key player in this space is Retrieval-Augmented Generation (RAG), a cutting-edge technology blending traditional AI with advanced information retrieval techniques.
š What is RAG?
Imagine you’re preparing a crucial market analysis report. You pull data from various sources ā sales figures, customer feedback, market trends. Now, envision an AI system doing this automatically, fetching relevant information to respond to complex queries, just like a supercharged search engine feeding into a smart AI assistant. That’s RAG in a nutshell.
RAG works in two steps:
1. Information Retrieval: Like a diligent researcher, it scans vast databases to find relevant data.
2. Intelligent Synthesis: It then integrates this data into its response, much like how an analyst would craft a report combining various data sources.
š¢ Applying RAG in Business Contexts
For businesses, RAG can be a game changer. It enhances customer service bots, making them not just conversational but also incredibly informed. In market analysis, it can provide richer insights by quickly referencing a wealth of data.
š§ But, There are Limitations
Data Quality is King: RAG’s output is only as good as its input. If your data sources are outdated or biased, so will be your AI’s conclusions.
Database Optimization: RAG needs well-organized, accessible databases. It’s not just about size but how well-structured and query-friendly your data repositories are.
Volume Constraints: While RAG is powerful, it’s not omniscient. In its current state of evolution, it can’t consume billions of data rows or process entire corporate databases in one go. Strategic data selection and prioritization become crucial.
š In Conclusion
RAG presents exciting possibilities for businesses, but it’s not a silver bullet. Success with RAG hinges on high-quality, well-managed data and realistic expectations about its capabilities. It’s a step towards smarter AI, but one that must be taken with careful planning, preparation, and understanding of its limits.
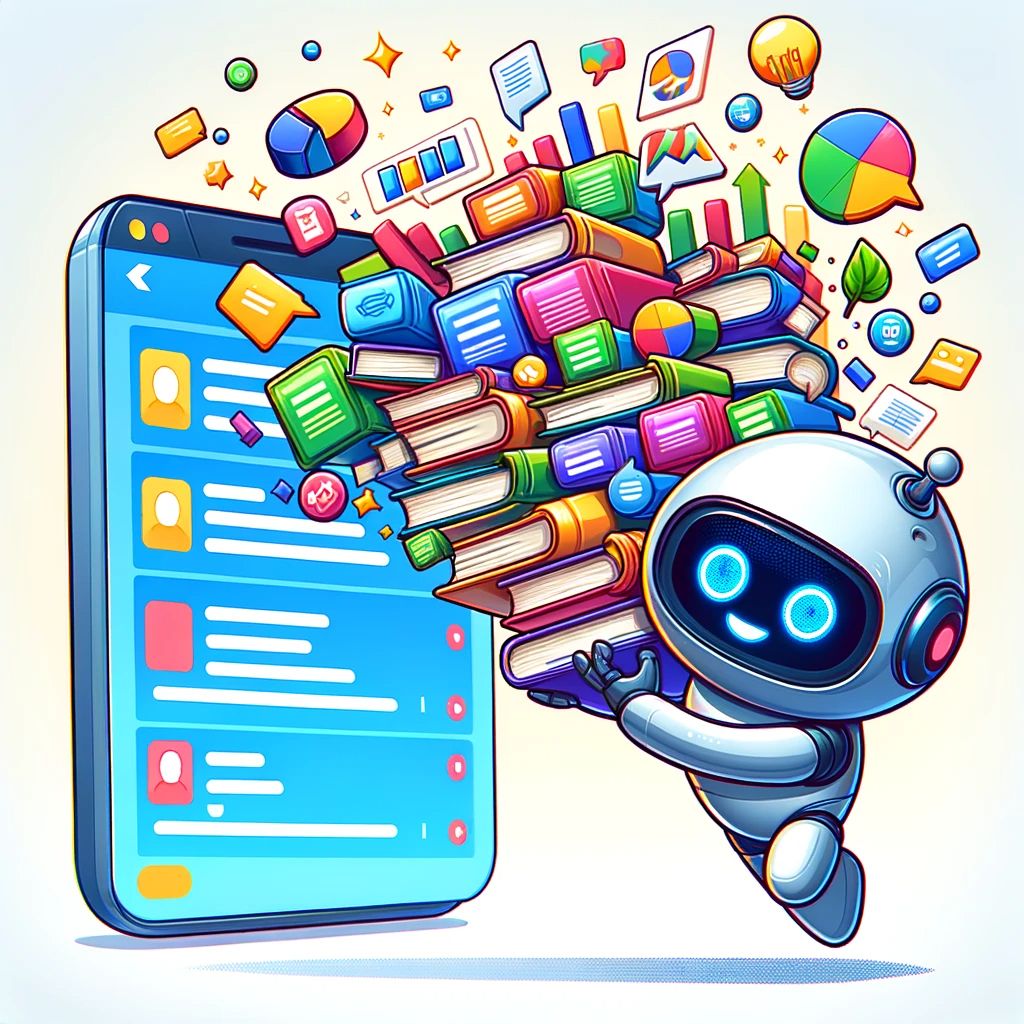