The advent of generative AI has ushered in a new era of innovation, disrupting industries and redefining what’s possible. From creative writing to coding and image generation, these models are pushing the boundaries of what artificial intelligence can achieve. However, as exciting as this technology is, its potential can only be fully realized through robust and scalable deployment strategies. This is where MLOps comes into play.
MLOps, short for Machine Learning Operations, is a set of practices and principles that enable the efficient and reliable deployment, monitoring, and maintenance of machine learning models in production environments. It’s a framework that ensures that models are not just accurate but also reliable, scalable, and maintainable.
In the context of generative AI, MLOps is particularly crucial for several reasons:
📈 Scalability: Generative AI models are often computationally intensive and require significant resources to operate effectively. MLOps provides the tools and processes to scale these models across multiple servers, clusters, or cloud environments, ensuring they can handle increasing demand and workloads.
👀 Continuous Monitoring: Generative AI models can be susceptible to drift, bias, and other issues that can impact their performance over time. MLOps enables continuous monitoring of model performance, allowing for early detection and mitigation of potential issues.
🐣 Reproducibility: The development and training of generative AI models often involve complex pipelines and intricate data processing steps. MLOps ensures that these pipelines are reproducible, making it easier to retrain or update models as needed.
📛 Governance and Compliance: As generative AI models become more prevalent, there will be increasing regulatory and compliance requirements around their development, deployment, and use. MLOps provides the necessary governance and auditing capabilities to ensure compliance with these regulations.
🤝 Collaboration: Generative AI models are often developed by multidisciplinary teams involving data scientists, software engineers, and domain experts. MLOps fosters collaboration by providing a shared set of tools, processes, and practices that enable seamless communication and integration across these diverse teams.
As organizations continue to embrace generative AI, implementing MLOps will be crucial for ensuring that these powerful models are deployed and maintained in a reliable, scalable, and responsible manner. By bridging the gap between data science and engineering, MLOps enables organizations to unlock the full potential of generative AI while mitigating risks and ensuring compliance.
In the rapidly evolving landscape of artificial intelligence, those who adopt MLOps practices will be well-positioned to stay ahead of the curve and harness the transformative power of generative AI.
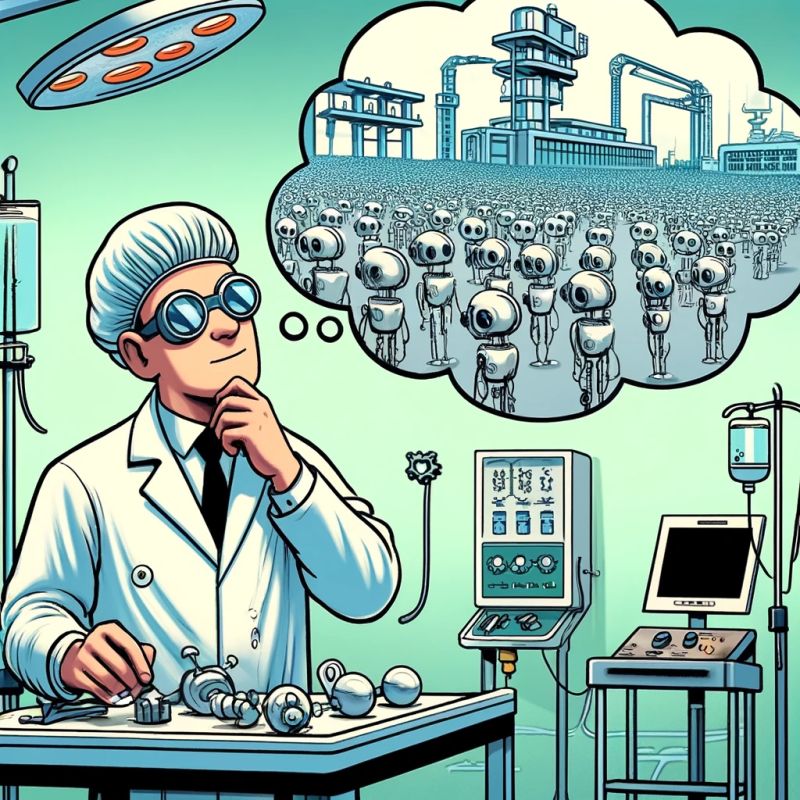