Bigger models, more data, and insane compute! Is this the ultimate formula for smarter AI, or have we reached the limit?
Ever wondered how foundational AI models like those from OpenAI and Anthropic got so smart, so fast? The answer lies in the “Scaling Hypothesis.” Simply put, the bigger the model, data and computational power, the better the AI. Think of it like a recipe: add more ingredients (parameters), stir in diverse data and supercharge with computing power. Voilà! Your AI model just leveled up.
The three key ingredients to the scaling hypothesis are …
⬆ Model Size: More parameters = better generalization and capabilities.
⬆ Training Data: Bigger, diverse datasets = better understanding and generalization.
⬆ Computational Resources: More power and time = deeper exploration and scaling.
Over the past few years the world has seen the proof that scaling works. Foundational models have demonstrated rapid emergent behaviors like arithmetic reasoning and common-sense knowledge through scaling.
But maybe it is not all cool guy emojis 😎 …
❓ What about Cost? Massive resources = high energy consumption and costs.
❓ What about training Data Limitations? Quality and quantity matter.
❓ Most importantly, what about Diminishing Returns? Do improvements slow down as models continue to scale?
Interestingly, recent rumors from OpenAI suggest that the latest models might not be seeing the same dramatic improvements as before. Is the scaling hypothesis reaching its limits?
What do you think? Can scaling alone lead to the golden artificial general intelligence (AGI), or will we need breakthroughs in algorithmic improvements and architectural innovations?
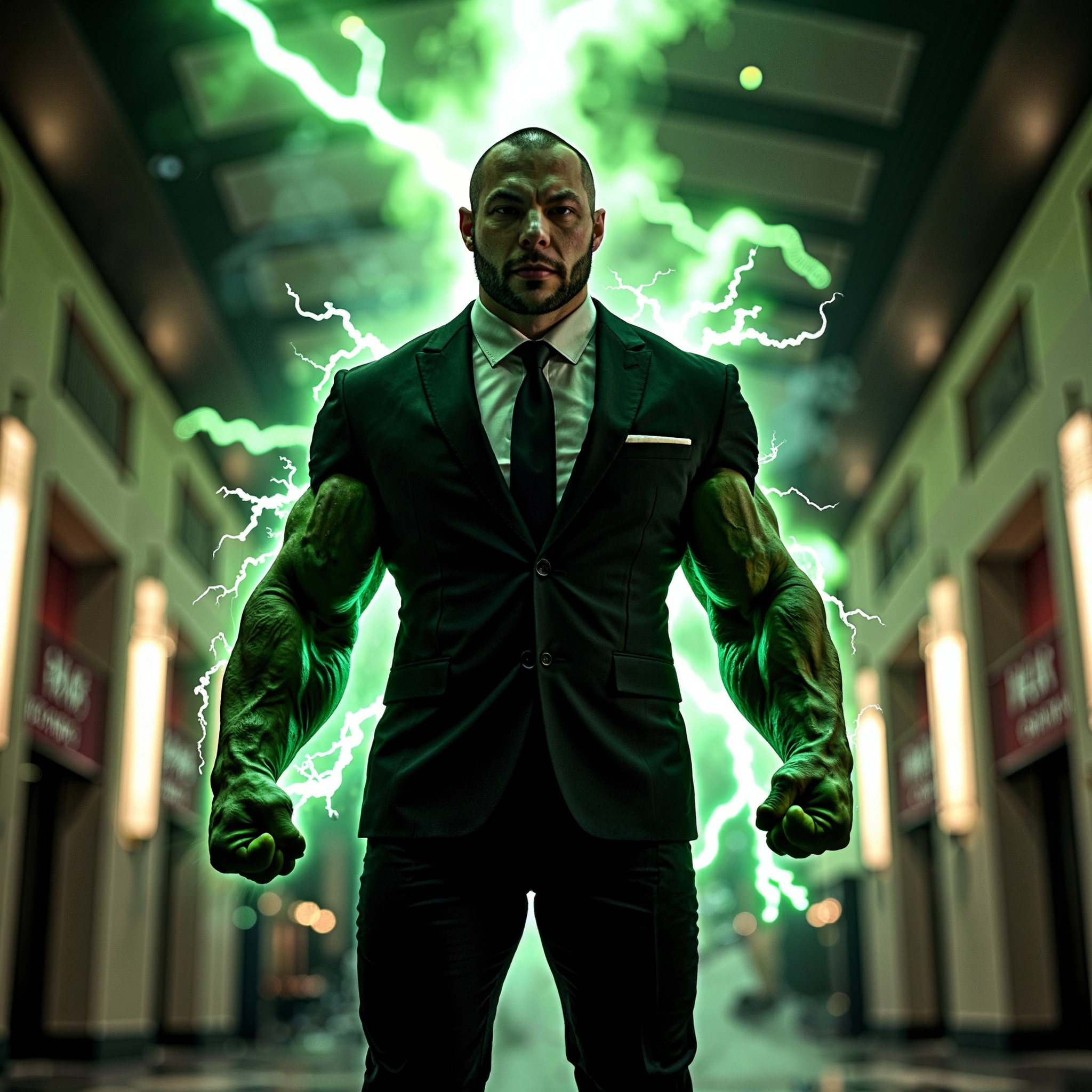