Generative AI is evolving fast, and if you’re leveraging Retrieval-Augmented Generation (RAG) like so many other organizations, it might be worth exploring the next potential evolution: Retrieval Interleaved Generation (RIG).
Besides one letter, whats the difference?
RAG retrieves information from external sources before generating a response. It collects relevant data upfront and then generates an answer based on that information. In contrast, RIG interleaves the retrieval process during the generation itself. Rather than pulling all the data in one go, it retrieves information continuously, adapting as it creates the response. This allows RIG to access fresh data mid-generation, making the output more relevant and context-aware.
The benefits of RIG are compelling. By weaving retrieval into the generation process, RIG can provide more precise and up-to-date responses, especially useful in dynamic environments where information is constantly changing. For industries dealing with real-time insights, like healthcare, finance, and customer service, RIG’s ability to refine its answers on the fly offers a significant edge. It also enables deeper insights for complex queries, where a simple upfront retrieval might not capture all the nuances.
However, RIG also comes with challenges. The computational requirements are higher since the model is retrieving data throughout the process, potentially leading to slower response times and higher costs. Additionally, training a system to balance generation and retrieval seamlessly is far more complex than standard RAG models. Without proper optimization, this could lead to longer delays or less efficient answers.
RIG could be an important advancement for organizations that need more adaptive, real-time AI systems, but implementing it effectively will require careful consideration of both the benefits and challenges.
Do you view RIG as a potential to push RAG to a thing of the past?
Full Research Paper:
https://lnkd.in/eFzt5wxN
Is it time for RAG to RIP? Googles improved RIG (Retrieval Interleaved Generation) has some potential.
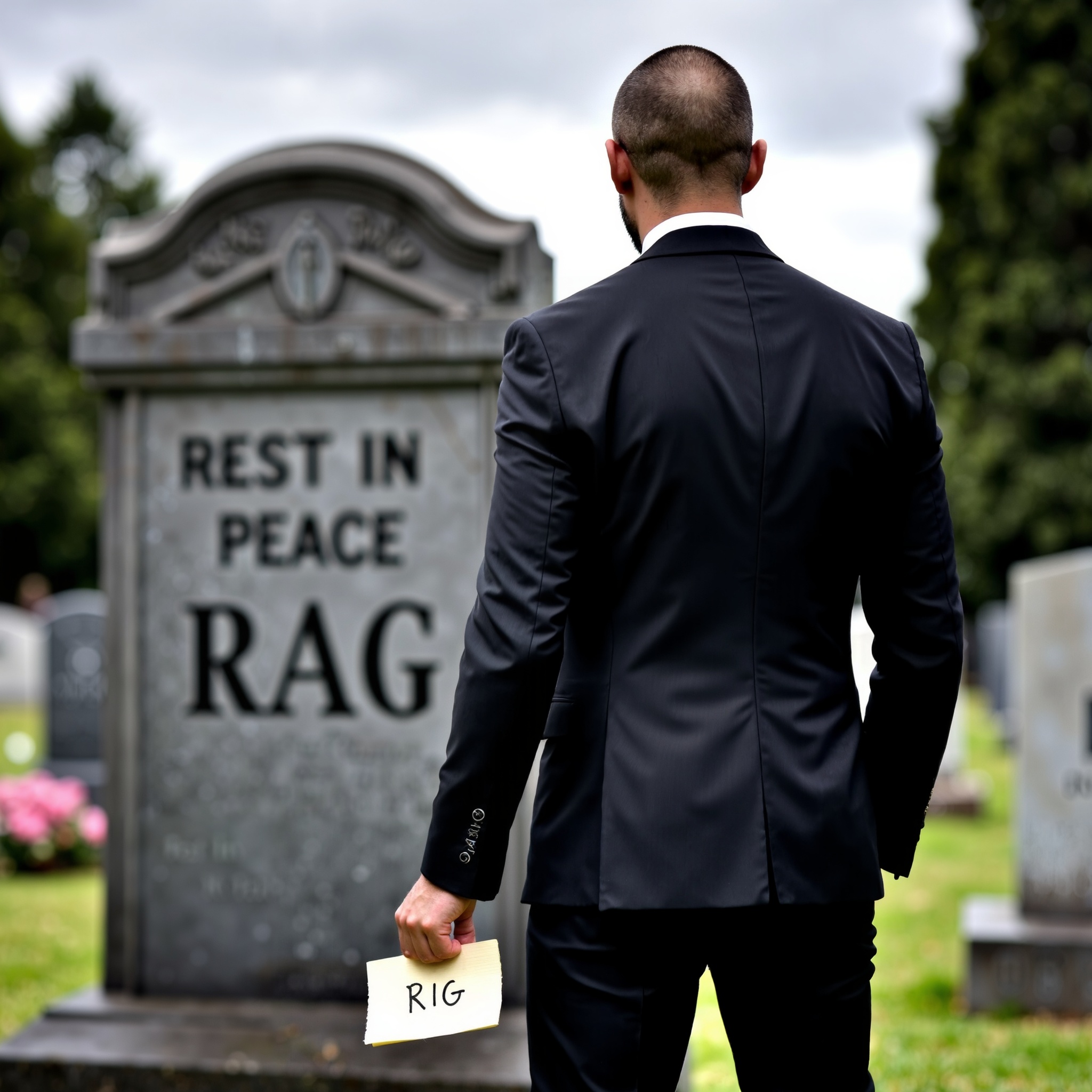