In the ever-evolving world of artificial intelligence, ensuring precision and reliability is paramount. As AI systems like large language models progress, one persistent challenge has been the occurrence of “hallucinations” – the presentation of plausible yet erroneous information. These inaccuracies can be misleading, especially when users expect factual responses.
A study by Meta AI presents a visionary solution to tackle this issue – the “Chain-of-Verification” technique. This innovation empowers language models to self-verify and rectify their own potential missteps.
Here’s how it works:
1️⃣ The model initially drafts a response to a query or prompt.
2️⃣ It then strategically plans a series of verification questions to fact-check its draft response, ensuring accuracy and integrity. For example, it may cross-check a date or event mentioned in the response.
3️⃣ The model independently answers these verification questions without peeking at its initial draft. This approach enhances the likelihood of providing precise and trustworthy short-form responses.
4️⃣ The model delivers a revised, verified response, thoughtfully incorporating any refinements based on the verification process.
In experiments, this self-verification technique showcased remarkable results, significantly reducing factual inaccuracies and hallucinations across various language tasks. Notably, the research found that models were more adept at answering targeted verification questions accurately than delivering long form responses.
This research sheds light on a way for AI systems to rigorously assess their own work. While it may not be perfect, self-directed verification and revision mark a significant stride toward making these models more reliable and trustworthy. This technique offers a promising route to bolster factual grounding without relying on external tools or human oversight.
As language models continually advance in capability, what other innovative methods will emerge to transform these powerful systems into truthful, benevolent assistants?
Research Paper:
Chain-of-Verification Reduces Hallucination in Large Language Models
Advancing AI Reliability: Through Self-Verification
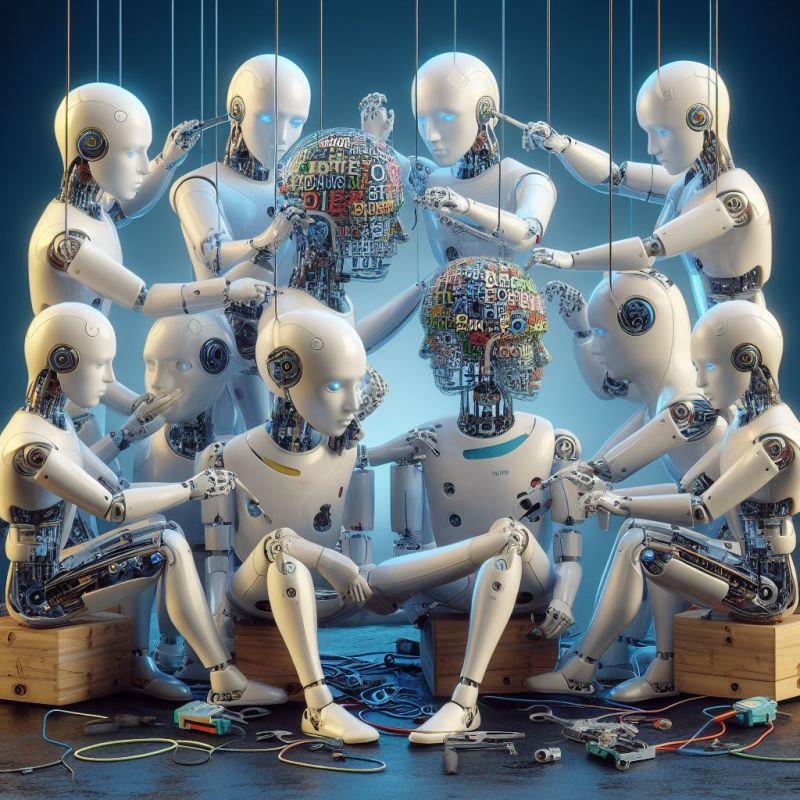